Taxis service offers
Taxis service offers great benefits and convenience to our daily life.However, in many cities, the taxi service supplies fail to meet the traveling demands especially in rush hours. To balance the supply anddemand, different measures had been executed in the last few years.On one hand, the authorities of many cities continuously estimatethe quantity of taxis and make dynamic adjustments in accordancewith the ever-increasing demand. On the other hand, some companiesprovide innovative services to connect passengers with drivers viabooking apps installed on mobile phones. These mobile booking appsbecome highly attractive around the world, since taxis are notoriouslydifficult to catch in metropolises.At first, these apps allowed users to bid for taxis by offering addi-tional bonus fees to drivers. This strategy could arouse the initiativeof drivers and make them become more active to serve passengers.The “invisible hand” would then help match the supply and demand.However, this bidding mechanism is clearly unfair for many peopleand was soon banned by the authorities.Along with the exponentially booming mobile payment market inChina, a new mechanism:money promotion, was implemented toreshape the supply and demand of taxis recently. For example, a battlebetween two Chinese taxi booking mobile apps: Didi [1] and Kuaidadi[2], had recently occurred in early 2014. These two apps, which arebacked by Internet giants Tencent and Alipay, gave promotion fees totaxi drivers for each deal made and also helped each taxi passengersave some money, when a customer had taken a taxi through the appand paid the fare through the mobile payment method.The hidden force in such battles is the players’ ambition to extendtheir territories in mobile payments, since mobile payment is one of themost important factor for any company wishing to dominate the era ofmobile Internet. Notice that money promotion for taxis booking is anefficient way to securing as many people as possible who use mobilepayments, the wrestling match between the rivals Tencent and Alibaba becomes more and more intensive
https://srisivasakthitravels.com/

Taxi drivers had highly welcomed this battle and had tried their bestto pick up every driver who is calling taxi through these apps. Thus,the taxi service pattern had been greatly changed during this battle.Many people began to complain that they cannot find a taxi to takeif they do not use such apps. Although these apps brings conveniencefor young high-tech people, they meanwhile make catching taxis moredifficult for senior citizens who are not familiar with mobile phone,foreign travelers who do not have a mainland Chinese bank account tolink up with the mobile payment system, and low-income citizens whocannot afford mobile phone or mobile service.Since March, 2014, the authorities of many Chinese cities had toset up new rules to ban the use of taxi booking apps during rushhour periods. For instance, the authorities of Shanghai halt the on-demand taxis booking mobile apps during morning peak (7:30A.M.to9:30A.M.) and evening peak (4:30A.M. to 6:30A.M.).However, a debate on whether such money promotion strategyshould be banned is not ended with such bans. The opinions fromdifferent people can be categorized into two groups.The first group of opinions claimed that the money promotion ben-efits the society, because the following changes optimize the suppliesof taxi serviceHypothesis 1) The money promotion may stir drivers to finish moretrips and serve more passengers so as to get more subsidies;Hypothesis 2) The money promotion may urge every driver toinstall taxis booking apps. These apps will help reduce the idletime of drivers and thus the waiting time of passengers.The second group of opinions claimed that the money promotionharms the society, because the following changes make the supplies oftaxi service more unfair:Hypothesis 3) The money promotion may cause drivers to choosethe passengers who travel shorter distances, since they couldserve more people and thus collect more subsidies;Hypothesis 4) The money promotion may make drivers stay in thecentral part of cities areas for the same reason
Call Taxi in Kumbakonam
https://goo.gl/maps/1rNVoifRFQ9snSor6
THE TAXI TRIP DATA
In this paper, we study the historical trip and fare logs of Beijingtaxis to investigate the change of taxi drivers’ behaviors under moneypromotion.Beijing is the capital of the People’s Republic of China and isone of the most populous cities around the world. Its populationhad grown over 21 000 000 in 2013. However, there are only nearly67 000 licensed taxis in Beijing, which cannot meet the ever increasingdemand of taxis service, especially in rush hours.The dataset used in this paper includes about 8.3 million taxi tripsthat made by over 9000 taxis during 40 days. Each trip record includesthe pickup and drop-off location and time, as well as anonymized taxilicense numbers. The personally identifiable information of passengershas been properly anonymized, so that we can only differ the driversbut not the passengers. Moreover, the names of the drivers are notreleased, either.The longitude and latitude location information in each taxi trip isobtained by converting the received GPS data into a planar coordinatesystem, since the latitudinal trend is not pronounced in the central partof Beijing city. The location errors caused by inaccurate GPS signalsare much smaller than the moving distances of taxi trips and are thusomitted in this paper.As pointed out in many studies, human traveling activities can beinfluenced by many factors. For example, human traveling patterns arenotably different in working-days and weekends/ holidays. Moreover,the weather also greatly affects the calling amount of taxi services. Toreduce the influences of such factors, we choose the sampling daysas Oct. 16–18, 21, 22, 24, 25, 28–31, Nov. 18–22, 25–27, 2013 andFeb. 17, 18, 20, 21, 24–28, Mar. 17–21, 24–28, 2014, respectively. Wecall the sampling days in 2013 the first time period, in which no moneypromotion was applied. The sampling days in 2014 is called the secondtime period, in which money promotion was applied. All these days areworking days and the weathers in these days are mild so that the traveldemands in these days are similar. Call Taxi in Kumbakonam
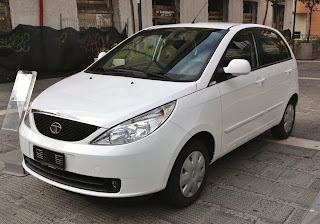
A battle between two Internet giants Tencent and Alipay had greatlystirred the taxis services during in early 2014. Although these twocompanies originally intended to extend their territories in mobilepayments by giving promotion fees to taxi drivers for each deal made,the money promotion had profoundly changed the pattern of taxisservices. Many people welcomed such promotion since they thoughttaxis drivers will become more diligent and available on-call. On thecontrary, many people found that they cannot find a taxi to take if theydo not use such apps.The authorities of some Chinese cities had to ban the use of taxibooking apps during rush hour periods, since they received increas-ing complaints from residents. However, no concrete data had beenreleased to explain the influence of money promotion, which makesthe ban somewhat unconvincing.In this paper, we study this problem based on the collected 40-daytrip data of over 9000 taxis in Beijing. Statistics show that the numberof taxis trips made by every vehicle per day increases under themoney promotion and the idle times become shorter, too. However,drivers preferred to pick up the passengers who travel shorter and thepassengers who went to the hot locations. In summary, the moneypromotion brings benefits to some but not all residents in the city.
During the review process of this paper, Didi and Kuaidadi hadannounced their official strategic combination on Feb. 14, 2015 (SaintValentine’s Day). We will study the influence of such a “marriage” inthe near future.This study also indicates that productively and critically employingbig data can help address long-standing questions of social justice,equity, and many other concerns [4], [17]. For example, Uber [18]is an international company which operates the mobile-app-basedtransportation world widely. It is preparing to penetrate into Chinesemarket recently. The above analysis results will be useful for such newdeveloping companies which aim to better share the markets and alsolocal governments which needs to guide the responses of residents tonew challenges. We believe the coming era of big data could provideus more chance to solve such formidable problems https://srisivasakthitravels.com/
https://goo.gl/maps/1rNVoifRFQ9snSor6
Battle Between Two Taxi Apps
A battle between two Chinese taxi booking mobile apps,namely, Didi and Kuaidadi, had recently occurred in early 2014. Thesetwo apps, which are backed by Internet giants Tencent and Alipay, gavepromotion fees to taxi drivers for each deal made and also allowed eachtaxi passenger to save some money, when a customer had taken a taxithrough the app and paid the fare through the mobile payment method.As expected, the taxi service pattern had been greatly changed during thisbattle. To address the debates on social justice, equity, and improvementsof taxi service, we collect 37-day trip data of over 9000 taxis in Beijing tostudy the influence of this pattern change. In the first 18 days, the battlehad not occurred and in the remaining 19 days, the battle is white-hot.We quantitatively demonstrate how several important service indices (e.g.,the traveling distances and idle time lengths) of taxi drivers had beenchanged. The spatial–temporal traveling patterns of taxis are then studied.Based on comprehensive analysis, the benefits and drawbacks broughtby money promotion are finally discussed. The obtained results indicatethat productively employing big data can help answer some importantquestions attracting the interest of the whole society.Sri Siva Sakthi Travels

In this paper, we study the historical trip and fare logs of Beijingtaxis to investigate the change of taxi drivers’ behaviors under moneypromotion.Beijing is the capital of the People’s Republic of China and isone of the most populous cities around the world. Its populationhad grown over 21 000 000 in 2013. However, there are only nearly67 000 licensed taxis in Beijing, which cannot meet the ever increasingdemand of taxis service, especially in rush hours.The dataset used in this paper includes about 8.3 million taxi tripsthat made by over 9000 taxis during 40 days. Each trip record includesthe pickup and drop-off location and time, as well as anonymized taxilicense numbers. The personally identifiable information of passengershas been properly anonymized, so that we can only differ the driversbut not the passengers. Moreover, the names of the drivers are notreleased, either.The longitude and latitude location information in each taxi trip isobtained by converting the received GPS data into a planar coordinatesystem, since the latitudinal trend is not pronounced in the central partof Beijing city. The location errors caused by inaccurate GPS signalsare much smaller than the moving distances of taxi trips and are thusomitted in this paper.As pointed out in many studies, human traveling activities can beinfluenced by many factors. For example, human traveling patterns arenotably different in working-days and weekends/ holidays. Moreover,the weather also greatly affects the calling amount of taxi services. Toreduce the influences of such factors, we choose the sampling daysas Oct. 16–18, 21, 22, 24, 25, 28–31, Nov. 18–22, 25–27, 2013 andFeb. 17, 18, 20, 21, 24–28, Mar. 17–21, 24–28, 2014, respectively. Wecall the sampling days in 2013 the first time period, in which no moneypromotion was applied. The sampling days in 2014 is called the secondtime period, in which money promotion was applied. All these days areworking days and the weathers in these days are mild so that the traveldemands in these days are similar.https://srisivasakthitravels.com/
https://goo.gl/maps/1rNVoifRFQ9snSor6
Performance Prediction Based on Historical Strategies
Taxi service strategies are key factors that influence therevenue performance of a taxi driver. Here, we show that it isfeasible to predict the performance of a taxi driver based on his/her historical strategies. We randomly split all the drivers infive groups. Again, we use four groups as the training set andthe remaining group as the test set. We use support vectorregression (libsvm [3]) to train a regression model on thedriver–strategy matrix of the training drivers. Then, we usethe obtained model to predict the revenue of a test driver inthe test data set based on their strategies. For a driverdi,ifthepredicted hourly revenue rate isyiand the true value isy′i, thenthe residual isri=|y′i−yi|. We use two metrics to evaluate theprediction error:
The mean residualr=mean(ri), which re-flects the average accuracy of the prediction, and the rel-ative residual of taxi drivers, which is computed as r=mean(ri/y′i), indicating the percentage of the mispredictionagainst the true value. A smaller value corresponds to a moreaccurate prediction result.The results in all the time slots are shown in Table III. Overall the time slots, we can obtain the residuals that are less than2.35 RMB/h, which suggests a quite accurate prediction result.The relative residuals in all the time slots except nighttime arequite small, which fully validate the feasibility of using theextracted taxi service strategies to predict taxi performance. Thereason of the relatively low prediction accuracy in nighttimeis probably because that taxi drivers may choose to sleep forsome time in late night, and this behavior influences the revenueperformance of the drivers

Taxi GPS traces are valuable resources to investigate taxidrivers’ service behaviors. In this paper, we have studied thetaxi drivers’ service strategies based on the activities of thou-sands of taxi drivers revealed in a real-life large-scale taxiGPS data set collected from 7600 taxis in Hangzhou, aimingto provide useful guidance to taxi drivers. To understand thebehaviors of a taxi driver, we first propose to separate the taxiGPS traces of each pair of shared taxi drivers based on twoobservations: 1) The shift handover location and time are moreor less fixed on most days; 2) the taxi generally stops for awhile in the shift handover location and shift handover timeslot for handover, with vacant status before and after the shifthandover event. We then convert each taxi delivery distance intocorresponding taxi revenue in each time slot and find that mostof the taxi drivers do not perform consistently well across dif-ferent time slots. Thus, we have proposed to study taxi drivers’behaviors in each time slot rather than on a daily basis.In this paper, we have proposed to model the taxi driver’sservice strategies from three perspectives, namely, passenger-searching strategies, passenger-delivery strategies, and service-area preference.
We further represented the service strategiesusing a feature matrix. In order to accurately identify thepassenger-searching strategies, we derived a method to learn theinitial intention of taxi drivers right after each drop-off event.Through measuring the correlation between each service strat-egy and the corresponding revenue, we reveal the efficient andinefficient strategies in each time slot and location. Generallyspeaking, it is found thathuntingis usually more efficient thanwaitingin order to find passengers locally, with a few excep-tions, such as in the airport where people go to fixed places totake taxis.Going distantbecomes a preferable service strategywhen a taxi drops off passengers in the suburb area, where taxisusually spend less time on average finding the next passengersby moving from nonhot areas to hot ones.https://srisivasakthitravels.com/
The correlation of thetaxi average passenger-delivery speed and the revenue showsthat, when the traffic becomes congested at busy time slots,choosing the light-traffic route increases the driver’s revenue.We also found that some service areas are more profitable thanothers for taxi drivers in different time slots of the day.Taxi service strategies are key factors influencing the taxidrivers’ revenue. Based on the extracted historical servicestrategies, we predict the performance of a taxi driver with asupport vector regression method. We obtain a residual of lessthan 2.35 RMB/h, suggesting that the extracted taxi servicestrategies with our proposed approach well characterize thedriving behavior and performance of taxi drivers.In the future, we plan to broaden and deepen this workin two directions. First, we plan to conduct further researchin characterizing subtle features of the taxi service behaviorsand strategies and to understand the human decision-makingprocess. Second, we attempt to explore the appropriate waysthat provide more concrete instructions to taxi drivers accordingto the taxi service strategies.
https://goo.gl/maps/1rNVoifRFQ9snSor6
DATA PREPROCESSING AND EMPIRICAL STUDY
We begin this section by introducing the taxi GPS tracescollected for our study. The large-scale taxi GPS data set wasacquired from approximately 7600 taxis served in Hangzhou, amegacity in China, for one year (April 2009 to March 2010).Each taxi is equipped with a GPS device that acquires the real-time taxi information, including its longitude/latitude, the timestamp, the passenger status (i.e., “occupied” or “vacant”), thedriving speed, and orientation. It uploads the data to a centralserver via a telecommunication network at a sampling rate ofonce per minute (i.e., the GPS sampling frequency is about onesample per minute). To avoid mistakes caused by device erroror network failure, we first filter out suspicious taxi records,such as taxi being vacant for the entire day, or lacking recordsfor extended periods of time, and obtain 6863 taxis to conductthe research.On a 2-D plane, a taxi’s moving trajectory over a timeinterval can be depicted by connecting the GPS points. Forinstance, Fig. 1 shows one taxi’s trajectory for a completepassenger-searching and passenger-delivery cycle, where redand blue lines correspond to the taxi’s vacant and occupiedstatuses, respectively. When the taxi is occupied, it is said tobe in the passenger-delivery stage; while the taxi is vacant, it isusually in the passenger-searching stage. The change from redto blue corresponds to a passenger pickup event, and the changefrom blue to red indicates a drop-off event.Before analyzing taxi driver’s service strategies, two datapreprocessing tasks need to be done.•Individual Taxi Driver GPS Trace Extraction: Almost allthe taxis in Hangzhou are served by two drivers, i.e., oneworks in daytime and the other in nighttime. As the shiftstatus of a taxi is not recorded in the taxi GPS traces, it isnecessary to detect when the shift handover takes place inorder to separate the taxi GPS traces of each driver. Onlywith each taxi driver’s GPS traces extracted, individualdrivers’ service strategies and revenues in different timeslots can be known.•Taxi Driver’s Performance Quantification: In order to dis-cover what service strategies are efficient and inefficient,we should be able to identify “good” and “ordinary”drivers by linking the service strategies with the revenuesgenerated. However, the taxi trip fares are not recordedin each taxi’s GPS traces; thus, it is necessary to quantifyeach taxi driver’s performance in each time slot accordingto the revenues calculated from the generated GPS traces.In the following sections, we will present how to deal withthe above two data preprocessing problems. We will also do anempirical study based on the processed data to visualize somestatistical results.
siva sakthi travels
For shared taxis, normally, the two drivers set up an agree-ment on when and where to hand over the taxi. Through interviews with taxi drivers in Hangzhou, we are told that theafternoon shift handover usually happens between 16:00 and18:00, and the morning shift handover takes place around06:00. The morning shift handover is generally more flexiblethan the one in the afternoon because many night shift driverspark the taxis at the agreed location around 03:00 so thatthe daytime drivers could take the taxis early if they wish.However, due to issues such as traffic, delay of last passenger deliveries, or personal reasons, it is hard to strictly obey the shifthandover agreement every day, particularly for the afternoonshift handover. Thus, the exact shift handover time detection becomes a nontrivial task in order to extract individual taxidriver’s GPS traces.https://srisivasakthitravels.com/
Taxi-Hunting Recommendation System
Recommender systems are constructed to search thecontent of interest from overloaded information by acquiring use-full knowledge from massive and complex data. Since the amountof information and the complexity of the data structure grow,it has become a more interesting and challenging topic to findan efficient way to process, model, and analyze the information.Due to the Global Positioning System (GPS) data recording thetaxi’s driving time and location, the GPS-equipped taxi can beregarded as the detector of an urban transport system. This pa-per proposes a Taxi-hunting Recommendation System (Taxi-RS)processing the large-scale taxi trajectory data, in order to providepassengers with a waiting time to get a taxi ride in a particularlocation. We formulated the data offline processing system basedon HotSpotScan and Preference Trajectory Scan algorithms. Wealso proposed a new data structure for frequent trajectory graph.Finally, we provided an optimized online querying subsystemto calculate the probability and the waiting time of getting ataxi. Taxi-RS is built based on the real-world trajectory data setgenerated by 12 000 taxis in one month. Under the condition ofguaranteeing the accuracy, the experimental results show that oursystem can provide more accurate waiting time in a given locationcompared with a naïve algorithm
RAJECTORY can be regarded as the trace of mobileobjects in space as times change.
Call Taxi in Kumbakonam
The convergence oftrajectory data allows the easy acquisition of information aboutthe trajectories of users using mobile devices [1]. Global Posi-tioning System (GPS), one of the growing technologies of ge-olocation, is widely used on taxis and makes the GPS-equippedtaxi be regarded as the detector of urban transport system. Itbecomes possible to access the location information of taxis ofa whole city at any time. Since the amount of trajectory data[3] and the complexity of data structure grow, it has become amore interesting and challenging topic to find an efficient wayto process, model, and analyze the mass data of movement.A taxi is an important tool, for people, to travel in the city,but sometimes it is common, for people, to face an awkward position [2]. Most people had such experience: you had to waitfor more than 10 min to get a taxi ride only because you were2 min late in the specific place. Another example: you had beenstanding by the street waiting for a cab for over 15 min, butyour neighbor just came out of his home, crossed the street,walked a few meters, and got a taxi ride immediately. It seemslike people have to collect more information to get a taxi rideinstead of longer hopeless waiting. Along with the increase inthe amount of taxis, it becomes urgent to solve recommendedlocation information and calculate waiting time by means oflarge-scale taxi GPS data.In the information age, it is very easy to collect in-formation. For the mentioned taxi problem, it becomes averyinterestingquestiontorecommendlocationinformationandcalculate waiting time by means of large-scale taxi GPS data.In this paper, we propose a processing method based on bigdata environment for the taxi GPS historical data, includingoffline and online processing. We implement a recommendationsystem called Taxi-hunting Recommendation System (Taxi-RS), and our system can calculate the probability and time ofgetting a taxi ride when giving information of time and point.The following are the major contents of this paper.1) We design data offline processing. First, we design analgorithm called HotSpotScan (HSS) algorithm to scanhotspots in a city. Then, the taxi preference trajectory isrecognized by a new algorithm called Preference Trajec-tory Scan (PTScan) algorithm.2) We propose an offline graph model by taxi preferencetrajectory and store offline compressed data by using amultiple adjacent table.3) Weconstructaprobabilitymodelbyusingtheofflinemodelto compute the probability and the waiting time of getting ataxi ride and analyze the complexity of online processing.The rest of this paper is organized as follows. Section IIgives a brief review on related literature. In Section III, someconception is formally introduced. The overview of the Taxi-RS is given in Section IV. In Section V, we introduce thekey algorithms of offline processing. In Section VI, we presentthe frequent trajectory graph (FTG) model. In Section VII, wepropose a calculation model to get a taxi ride. The results of theexperimental evaluation are given in Section VIII. Remarks arestated under the conclusion https://srisivasakthitravels.com/
https://www.facebook.com/Sri-Siva-sakthi-Travels-102008227873939/
https://goo.gl/maps/1rNVoifRFQ9snSor6
Development of Traveling
A W band folded waveguide Traveling Wave Tube that adopts horseshoe-shaped attenuator and a periodic step-tapering folded waveguide slow wave structure is reported in this paper. In this Traveling Wave Tube, Reflection from severability was effectively reduced and self-oscillation was depressed, and also electronic efficiency is raised by about 1.5~2% comparing with uniform periodic folded waveguide Traveling Wave Tube.
Because of the merits of all metal structure, simple coupling structure and compatibility with micro-fabrication technology, folded waveguide slow wave structure is widely used in millimeter and Terahertz Traveling Wave Tube, but suppression of self-oscillation and improvement of interaction efficiency have been the two most important questions[1-4]. Self-oscillation in W band folded waveguide Traveling Wave Tube commonly includes reflection oscillation, backward-wave oscillation and band-edge oscillation. Reflection oscillation is mainly caused by individual frequency mismatch in its wide frequency band due to machining error and welding deviation of TWT, backward wave oscillation will occur when resonant condition is satisfied between electrical parameters and interaction length, and the band-edge oscillation is due to the mismatch of slow wave structure’s ends. Therefore, it is verynecessary to improve processing precision of the folded waveguide and optimize concentrated attenuator and periodic distribution of folded waveguide slow wave structure to suppress the oscillation and improve the interaction efficiency. Sri Siva Sakthi Travels

This W-band folded waveguide Traveling Wave Tube adopts a horseshoe-shaped attenuator shown in Fig.1 and simulation result by HFSS is shown in Fig.2. From Fig.2, we can see that voltage standing wave ratio in wide range of 10GHz are less than 1.1. In order to improve interaction efficiency of the folded waveguide Traveling Wave Tube, a periodic step-tapering folded waveguide slow wave structure was designed in Fig.3. Compared with uniform periodic folded waveguide, interaction efficiency increased from 3% to 5%. Simulation results by CST are shown in Fig.4 and Fig.5, a stable output power and pure peak spectrum can be seen from above figures A low VSWR energy transfer system was fabricated and combined with the periodic step-tapering folded wave guide SWS for cold measurement. The test results are shown in Fig.6. From Fig.6, we can see that voltage standing wave ratio in 10GHz broadband range are less than 1.367.W-band folded wave guide periodic step-tapering Traveling Wave Tube is shown in Fig.7 and test electrical parameters are listed in table 1. Fig.8 shows that there is no oscillation power when input power is zero and Fig.9 shows that there are two peaks when input power is not zero, right peak is true power output point and left for its image produced by mixing. Fig.10 shows output power increases with input power and the maximum output power is 65W with electronic efficiency is about 4%.
A horseshoe shaped attenuator and a periodic step tapering folded waveguide slow wave structure can not only effectively suppress self-oscillation, but also raise interaction efficiency by 1.5~2%, which laid a good foundation for stable and efficient work of a W-band Traveling Wave Tube. https://srisivasakthitravels.com/
https://www.facebook.com/Sri-Siva-sakthi-Travels-102008227873939/